Accelerating Scientific Workflows with LLM Agents
Large Language Model (LLM) agents are transforming the way we approach the Design, Make, Test, and Scale (DMTS) steps in chemistry research. These AI-driven collaborators can extract insights from literature, model reaction conditions, and analyze data with unprecedented speed.
Example Reaction and Corresponding Prompts
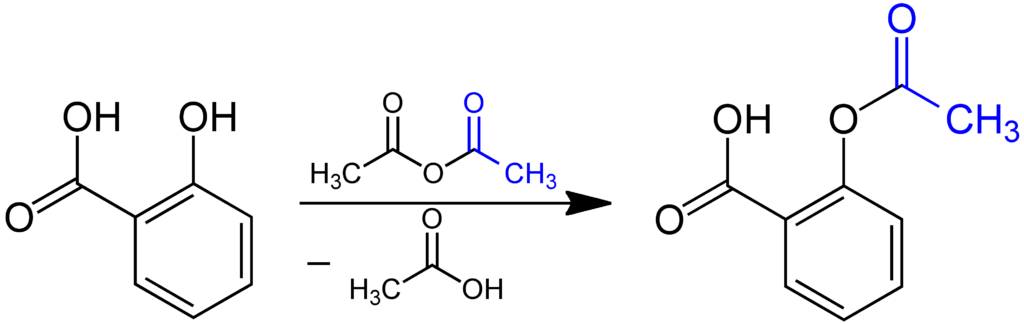
Acetylsalicylic acid is synthesized via the esterification of salicylic acid with acetic anhydride, catalyzed by sulfuric or phosphoric acid under reflux. The reaction follows a nucleophilic acyl substitution mechanism, yielding aspirin and acetic acid as a byproduct. Purification is typically achieved through recrystallization from water or ethanol to remove unreacted reagents and side products.
25 Prompts to Guide the Process (DMTA)
Prompt | Relevant LLM Agent |
„What are the known synthetic pathways for aspirin?“ | Literature Agent |
„What reaction conditions yield the highest purity for aspirin synthesis?“ | Computational Chemistry Agent |
„What side products should be considered when synthesizing aspirin?“ | Cheminformatics Agent |
„How do different catalysts affect aspirin synthesis?“ | Computational Chemistry Agent |
„What solvent effects influence aspirin purity?“ | Computational Chemistry Agent |
„What are the common impurities found in synthesized aspirin?“ | Research Data Agent |
„What is the impact of reaction temperature on yield?“ | Computational Chemistry Agent |
„How can process safety be improved for aspirin synthesis?“ | Research Data Agent |
„What are the industrial best practices for aspirin synthesis?“ | Literature Agent |
„How do regulatory guidelines impact aspirin production?“ | Literature Agent |
„What are the standard analytical methods for aspirin quality control?“ | QA/QS Agent |
„How does aspirin synthesis scale from lab to production?“ | Scale-Up Agent |
„What are the cost implications of different reaction pathways?“ | Research Data Agent |
„How do impurities affect aspirin’s stability?“ | QA/QS Agent |
„What are the standard safety measures for aspirin production?“ | Research Data Agent |
„How does aspirin synthesis compare using batch vs. continuous reactors?“ | Scale-Up Agent |
„What are the environmental impacts of aspirin synthesis?“ | Research Data Agent |
„What factors influence the shelf-life of aspirin?“ | QA/QS Agent |
„How does RDKit model aspirin reaction kinetics?“ | Cheminformatics Agent |
„What experimental results exist for aspirin synthesis?“ | Research Data Agent |
„How does ORCA predict aspirin molecular behavior?“ | Computational Chemistry Agent |
„What LIMS data is available for past aspirin synthesis experiments?“ | Research Data Agent |
„What improvements does IBM RXN suggest for aspirin synthesis?“ | Synthesis Agent |
„How do different acetylating agents impact aspirin yield?“ | Computational Chemistry Agent |
„What are the latest patents related to aspirin synthesis?“ | Literature Agent |
Although not every agent from the example is fully productive yet, each one contributes targeted expertise that drives efficiency. By combining literature analysis, computational chemistry, and data insights, researchers gain a powerful toolset to streamline a development of aspirin synthesis. The following curated list of prompts illustrates how LLM agents can pave the way for a more robust and scalable DMTS process.